AI Stock Pickers: Do They Beat the Market, or Just Look Good on Paper?
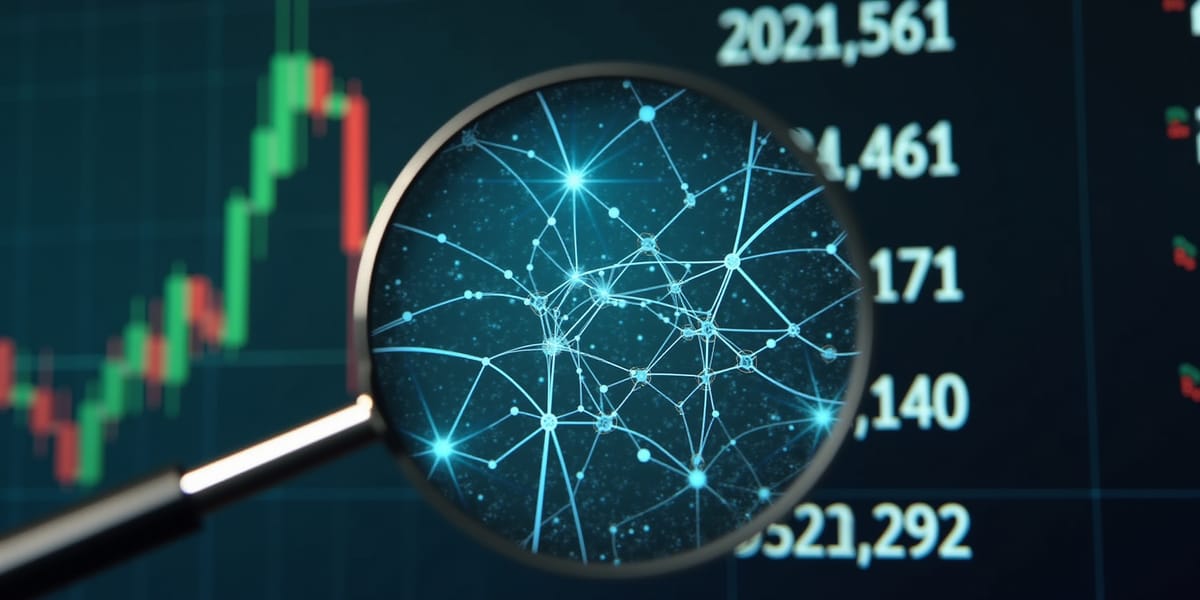
Large Language Models (LLMs) are the new wizards of Wall Street, or so the story often goes. We’ve seen a flurry of research suggesting these AI powerhouses can digest vast amounts of financial data – news, company filings, market sentiment – and make savvy investment decisions. But a new study from researchers at the University of Edinburgh, Sungkyunkwan University, UCLA, and the University of Oxford urges a healthy dose of skepticism, suggesting that when it comes to the long game, these AI investors might not be outperforming the market as consistently as some initial reports claim.
The paper, titled "Can LLM-based Financial Investing Strategies Outperform the Market in Long Run?," takes a critical look at how LLM-based trading strategies are typically evaluated. The authors, Weixian Waylon Li, Hyeonjun Kim, Mihai Cucuringu, and Tiejun Ma, argue that many current assessments are conducted over narrow timeframes and with a limited selection of stocks. This, they contend, can lead to overly optimistic results, often inflated by what are known in the financial world as survivorship bias (only looking at stocks that "survived"), look-ahead bias (using future information accidentally), and data-snooping bias (finding patterns that aren't really there by testing too many strategies on the same data).
To address these shortcomings, the researchers developed FINSABER, a more rigorous backtesting framework. It's like putting LLM investment strategies through a much tougher, longer obstacle course. FINSABER evaluates strategies over two decades, across more than 100 symbols, and systematically accounts for those pesky biases. The core idea is to see if the LLMs' shine holds up under conditions that more closely resemble the messy reality of long-term investing.
The findings are quite revealing. When subjected to FINSABER’s scrutiny, the previously reported advantages of LLM-based strategies "deteriorate significantly." It turns out that picking a handful of historically successful stocks (like Tesla or Amazon) for a short evaluation period can make an LLM look like a genius. But expand the timeframe and the stock universe, and the picture changes. The study also found that the choice of the underlying LLM itself (say, GPT-4 versus a smaller variant) dramatically impacts performance, and bigger isn't always better, hinting that some reported successes might be due to cherry-picking models or setups.
Perhaps most interestingly, the market regime analysis showed a distinct pattern: LLM strategies tended to be overly conservative during bull markets, missing out on potential gains, and then overly aggressive in bear markets, leading to heavier losses. This suggests a lack of sophisticated risk control and an inability to adapt to changing market weather. As the authors put it, these LLMs need to get better at "trend detection and regime-aware risk controls over mere scaling of framework complexity."
This research doesn't mean LLMs have no future in finance. Instead, it’s a crucial reality check. The "aha!" moment here isn't that LLMs can process text, but that truly outperforming the market requires more than just understanding news sentiment. The study highlights that traditional, often simpler, investment strategies frequently held their own or even outperformed the LLM approaches in these more robust tests.
The implications are clear: for LLMs to become truly reliable financial advisors or autonomous traders, the focus needs to shift from simply making them more complex to making them smarter about market dynamics and risk. As the paper concludes, future development should prioritize enhancing uptrend detection and embedding dynamic, regime-aware risk controls. It’s a call for more intellectual honesty in evaluation and a more nuanced approach to building AI that can navigate the complexities of the financial world, not just for a quarter, but for the long haul.