How AI Models Are Helping Us Understand Language in the Brain
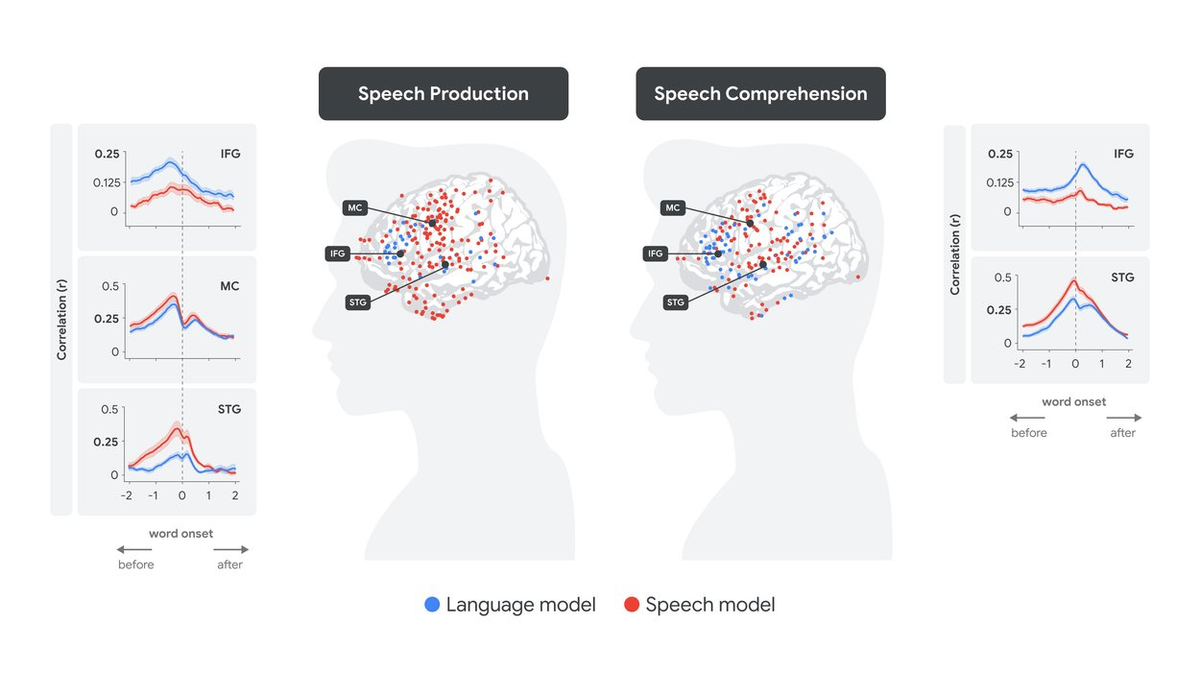
How does the intricate dance of neurons in our brain allow us to effortlessly chat, understand stories, or plan what we're going to say next? For decades, scientists have sought models to explain this remarkable feat. Traditionally, linguistic theories focused on symbolic rules, like grammar trees. But the rise of large language models (LLMs), like those powering chatbots, offers a radically different approach. These AI systems learn the statistical patterns of language from vast amounts of text, creating complex internal representations – often called embeddings – without explicit grammatical rules.
Now, a fascinating line of research suggests these AI models might be more than just powerful tools; they could be mirrors reflecting some fundamental aspects of how our own brains process language. A collaboration between researchers at Google Research, Princeton University, NYU, and the Hebrew University of Jerusalem has been exploring this connection, culminating in a recent study published in Nature Human Behaviour. Their findings reveal striking parallels between the internal workings of an AI speech-to-text model and the neural activity observed in human brains during natural conversation.
The researchers analyzed brain activity recorded directly using intracranial electrodes from individuals engaged in spontaneous conversations. They then compared these neural signals, word by word, to the internal "embeddings" generated by Whisper, an open-source speech-to-text AI model. Think of these embeddings as unique digital fingerprints the AI creates for speech sounds and word meanings based on context. The team looked at two types: speech embeddings (representing the sound qualities) from the AI's encoder and language embeddings (representing meaning and context) from its decoder.
The results were compelling. As described in their original research post, when participants listened to speech, their brain activity first aligned strongly with the AI's speech embeddings in auditory processing regions (like the superior temporal gyrus, or STG). A few hundred milliseconds later, activity in higher-level language areas (like Broca's area in the inferior frontal gyrus, or IFG) aligned with the AI's language embeddings, corresponding to the brain decoding the meaning.
Intriguingly, the sequence reversed during speech production. Before a word was spoken, activity in language areas (IFG) matched the language embeddings, reflecting planning what to say. Shortly after, activity in the motor cortex aligned with speech embeddings, corresponding to planning how to articulate the sounds. Finally, after the word was spoken, auditory areas (STG) again matched speech embeddings, likely reflecting the brain monitoring its own output.
This alignment suggests that while the AI wasn't designed based on brain architecture, its method of processing sound and meaning mirrors the functional flow of information in the human brain during conversation. It also revealed a "soft hierarchy" – language areas primarily process meaning but still track sound features, while auditory areas focus on sound but retain some meaning information. Further studies by the team, published in Nature Neuroscience and Nature Communications, found that both brains and LLMs seem to rely on predicting upcoming words and that the "geometry" of how LLMs relate words is similar to how the brain does.
However, the parallels aren't perfect. The underlying architecture differs significantly. Transformer models process large chunks of text simultaneously, whereas the brain appears to handle language more sequentially and recurrently, word by word.
Despite these differences, the research demonstrates that LLMs provide a powerful new framework for neuroscience. By comparing AI representations to brain activity, scientists can gain unprecedented insights into the neural code for language. It's a compelling example of how studying artificial intelligence can deepen our understanding of natural intelligence, potentially paving the way for both better AI and a clearer picture of the human mind at work.